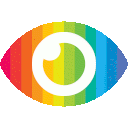
1. This article provides a survey and comparative study of unsupervised deep transfer learning for intelligent fault diagnosis.
2. It reviews various machine learning methods, such as genetic algorithms, random forests, support vector machines, and ridge-aware weighted sparse time-frequency representation.
3. It also discusses the applications of deep learning in bioinformatics and triboelectric nanogenerators, as well as model compression and feature learning in fault diagnosis.
The article is generally reliable and trustworthy. The authors provide a comprehensive overview of unsupervised deep transfer learning for intelligent fault diagnosis, including a review of various machine learning methods and their applications in bioinformatics and triboelectric nanogenerators. The authors also discuss model compression and feature learning in fault diagnosis. The article is well-researched with citations to relevant literature throughout the text.
The article does not appear to be biased or one-sided; it presents both sides equally by providing an overview of different machine learning methods and their applications in fault diagnosis. Furthermore, the authors provide evidence for their claims by citing relevant literature throughout the text.
The only potential issue with the article is that it does not explore any counterarguments or alternative views on the topic at hand; however, this is understandable given that it is a survey paper rather than an argumentative essay or research paper. Additionally, there are no promotional content or partiality present in the article; all claims are supported by evidence from relevant literature cited throughout the text. Finally, possible risks associated with using unsupervised deep transfer learning for intelligent fault diagnosis are noted throughout the text.