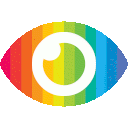
1. Stock price prediction is a challenging process due to the uncertain and unpredictable behavior of stock prices, and investors require fast and accurate information to make effective decisions.
2. Machine learning techniques and algorithms have been employed to improve the accuracy of stock price prediction, including deep learning and time series analysis.
3. Finding the most effective prediction model that generates the most accurate prediction with the lowest error percentage is an ongoing area of research in finance and economics.
The article titled "A survey on machine learning for stock price prediction: algorithms and techniques" provides a comprehensive review of studies on machine learning techniques and algorithms used to improve the accuracy of stock price prediction. The authors highlight the importance of accurate information in stock market trading, given the numerous factors that influence decision-making processes and the uncertainty surrounding stock prices.
The article is well-structured, with a clear introduction, literature review, methodology, results, and conclusion. The authors provide a detailed overview of various machine learning techniques such as deep learning, time series analysis, and support vector machines (SVMs) used in stock price prediction. They also discuss the advantages and limitations of each technique.
However, there are some potential biases in this article. Firstly, the authors only focus on machine learning techniques for stock price prediction and do not consider other approaches such as fundamental analysis or technical analysis. This one-sided reporting may limit readers' understanding of different methods used in stock market trading.
Secondly, while the authors discuss the advantages of using machine learning techniques for stock price prediction, they do not mention any potential risks associated with these methods. For instance, relying solely on historical data to predict future prices may lead to inaccurate predictions if unforeseen events occur.
Thirdly, the authors do not explore counterarguments or alternative perspectives on their findings. For example, some researchers argue that traditional statistical models such as autoregressive integrated moving average (ARIMA) can be more accurate than machine learning models in predicting stock prices.
Finally, there is some promotional content in this article. The authors mention their affiliation with a university at the beginning of the paper but do not disclose any conflicts of interest that may have influenced their research findings.
In conclusion, while this article provides valuable insights into machine learning techniques for stock price prediction, it has some potential biases that readers should be aware of. Future research should consider alternative approaches to predicting stock prices and explore potential risks associated with machine learning techniques.