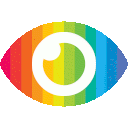
1. Clean energy stock prices are becoming increasingly important to investors due to climate change, green consumers, and technological innovation.
2. Machine learning methods, particularly random forests, offer better accuracy in predicting stock prices compared to regression-based approaches or technical indicators alone.
3. Random forests have been underutilized in the literature on stock price prediction despite their high accuracy in predicting stock price direction.
The article "A Random Forests Approach to Predicting Clean Energy Stock Prices" discusses the use of machine learning methods, specifically random forests, for predicting the stock prices of clean energy companies. The article highlights the increasing interest in investing in clean energy companies due to climate change, green consumers, and technological innovation. However, there is a lack of information on predicting clean energy stock prices.
The article acknowledges the difficulty in predicting stock prices and cites the prevailing wisdom that stock prices are unpredictable. It also notes that regression-based approaches or technical indicators provide mixed results, while machine learning methods offer better accuracy. The article provides examples of papers that have used random forests for stock price prediction and highlights their high accuracy.
The purpose of this paper is to predict clean energy stock price direction using random forests with a multi-step forecast horizon. The analysis uses several well-known technical indicators for feature selection and compares the forecasting accuracy of logit models, bagging decision trees, and random forests.
Overall, the article provides a comprehensive overview of using machine learning methods for predicting clean energy stock prices. However, there are some potential biases and missing points of consideration in the analysis. For example, the article does not discuss any potential risks associated with investing in clean energy companies or acknowledge any counterarguments against investing in these companies.
Additionally, while the article acknowledges that momentum and economic or psychology behavior factors may contribute to stock price predictability, it does not explore these factors further or consider their impact on clean energy stocks specifically. This could potentially limit the applicability of the analysis to other industries or sectors.
Furthermore, while the article provides examples of papers that have used random forests for stock price prediction and highlight their high accuracy compared to other machine learning methods like artificial neural networks or support vector machines, it does not provide a clear explanation as to why more complicated methods are preferred over simpler ones like random forests.
In conclusion, while "A Random Forests Approach to Predicting Clean Energy Stock Prices" provides valuable insights into using machine learning methods for predicting clean energy stock prices, it could benefit from a more balanced discussion of potential risks and counterarguments and a clearer explanation of why more complicated methods are preferred over simpler ones.