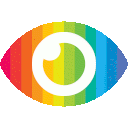
1. Falls are a frequent reason for deaths or post-traumatic complications in the elderly, making early detection crucial for survival and necessary support.
2. A scalable architecture of a system that can monitor thousands of older adults, detect falls, and notify caregivers has been developed using machine learning models.
3. The system was tested on both cloud-based data centers and edge IoT devices, with results showing significant reduction in the size of stored and transmitted data while performing fall detection on the edge.
The article "Fall detection in older adults with mobile IoT devices and machine learning in the cloud and on the edge" presents a scalable architecture for fall detection in older adults using mobile IoT devices and machine learning. The article highlights the importance of remote monitoring of older adults and detecting dangers in their health, particularly falls, which can be life-threatening or result in severe health problems.
The article provides a comprehensive review of related works on fall detection, categorizing them based on the device used and the method used for detecting falls. The authors also discuss the limitations of existing solutions, such as high variability for different groups of monitored people and lack of scalability.
The authors present their solution, which relies on machine learning-based methods for fall detection. They tested several ML models and found that Boosted Decision Trees resulted in the best classification performance. They also experimentally tested fall detection on both a cloud-based data center and an Edge IoT device, showing that significant reduction in data transmission can be achieved by performing fall detection on the Edge.
Overall, the article provides valuable insights into fall detection using mobile IoT devices and machine learning. However, there are some potential biases and missing points of consideration that should be noted.
Firstly, while the article acknowledges that falls can occur during sporting activities, it primarily focuses on falls among older adults. This may lead to a bias towards this specific group rather than considering falls as a broader issue affecting people across all age groups.
Secondly, while the authors discuss various solutions for fall detection based on wearable devices with sensors or external devices like cameras, they do not explore potential privacy concerns associated with these solutions. For example, wearable devices may collect sensitive personal information about individuals' health status or daily activities.
Thirdly, while the authors present their solution as scalable for monitoring thousands of older adults simultaneously, they do not discuss potential risks associated with large-scale data collection or storage. For example, there may be security risks associated with storing sensitive health data in the cloud or on IoT devices.
In conclusion, while the article provides valuable insights into fall detection using mobile IoT devices and machine learning, it is important to consider potential biases and missing points of consideration. Further research is needed to explore potential privacy and security risks associated with large-scale data collection and storage for fall detection.