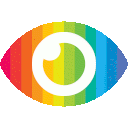
1. Network representation learning (NRL) aims to learn low-dimensional vector representations of a network for tasks such as node classification and link prediction.
2. Existing NRL methods often focus on the local structure of the network, but sparsity is a common problem in complex networks. Community structure can be utilized to supplement the sparseness of the structure.
3. The proposed Community Aware and Relational Attention (CARA) method combines community aware random walks with a two-layer attention mechanism to enhance network representation learning by capturing both global structure and semantic relationships between nodes. Experimental results show its superiority compared to other state-of-the-art methods.
本文是一篇关于网络表示学习的论文,提出了一种名为CARA的方法来增强网络表示学习。文章首先介绍了网络表示学习的背景和意义,并指出现有方法存在的问题。然后,作者提出了CARA方法,并详细介绍了其原理和实验结果。
然而,本文存在以下几个问题:
1. 偏重技术细节而忽略社会影响
本文主要关注技术方面,忽略了网络表示学习对社会的影响。例如,在社交网络中使用这种方法可能会导致用户数据被滥用或泄露。
2. 缺少对其他领域研究成果的引用
本文没有引用其他领域相关研究成果,如社会科学、心理学等。这些领域的研究成果可以帮助我们更好地理解网络结构和节点之间的关系。
3. 实验数据来源不够广泛
本文只使用了四个真实世界网络进行实验,样本量较小且覆盖范围有限。因此,该方法在其他类型或规模更大的网络上是否有效仍需进一步验证。
4. 没有考虑到潜在风险
本文没有探讨使用该方法可能带来的潜在风险,如数据隐私泄露、算法歧视等。这些问题需要被认真考虑和解决。
综上所述,本文提出的CARA方法在技术方面具有一定的创新性和实用性,但作者应该更加关注其社会影响和潜在风险,并引用更多相关研究成果来支持其主张。