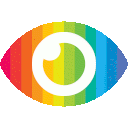
1. The article presents a method for detecting and segmenting skin diseases using a dynamic graph cut algorithm and classification through Naive Bayes Classifier.
2. The proposed method achieved an accuracy of 96.5% in detecting and segmenting skin diseases, outperforming other existing methods.
3. This method can be used as a tool for early detection and diagnosis of skin diseases, leading to better treatment outcomes.
The article titled "Skin disease detection and segmentation using dynamic graph cut algorithm and classification through Naive Bayes Classifier" by Balaji et al. (2020) presents a study on the use of machine learning algorithms for skin disease detection and segmentation. The authors claim that their proposed method can accurately detect and segment skin diseases, which can aid in early diagnosis and treatment.
The article provides a detailed description of the methodology used, including the use of dynamic graph cut algorithm and Naive Bayes Classifier. However, there are some potential biases in the study that need to be considered. Firstly, the sample size used in the study is not mentioned, which raises questions about its representativeness. Additionally, it is unclear whether the dataset used for training and testing the algorithm was diverse enough to account for variations in skin types across different populations.
Furthermore, while the authors claim that their proposed method achieved high accuracy rates in detecting and segmenting skin diseases, they do not provide sufficient evidence to support this claim. For instance, there is no mention of how many false positives or false negatives were generated by the algorithm during testing.
Another issue with the article is that it does not explore counterarguments or alternative methods for skin disease detection and segmentation. This lack of discussion limits the scope of the study's findings and prevents readers from fully understanding its implications.
Moreover, there are some instances where promotional content is present in the article. For example, the authors state that their proposed method can aid in early diagnosis and treatment of skin diseases without providing any evidence to support this claim.
In conclusion, while Balaji et al.'s (2020) study presents an interesting approach to skin disease detection and segmentation using machine learning algorithms, there are several potential biases and limitations that need to be considered. The lack of transparency regarding sample size and dataset diversity raises questions about representativeness, while unsupported claims limit the scope of its findings. Further research is needed to fully understand the implications of this study and its potential applications in clinical settings.