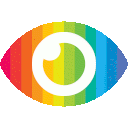
1. A digital image correlation (DIC) based surface strain monitoring technique is proposed for pixel-level classification of DIC strain field images.
2. A DeepLabv3+ encoder-decoder architecture combined with different feature extraction networks is investigated.
3. The prediction accuracy of the semantic segmentation model trained from the FE data is comparable with that of the model trained from the experimental data, demonstrating that the proposed machine learning approach for DIC measurement is cost-effective.
The article “Digital Image Correlation (DIC) Based Damage Detection for CFRP Laminates by Using Machine Learning Based Image Semantic Segmentation” provides a comprehensive overview of a new approach to structural health monitoring (SHM). The article presents a convolutional neural network (CNN) based image semantic segmentation technique for pixel-level classification of DIC strain field images, and investigates a DeepLabv3+ encoder-decoder architecture combined with different feature extraction networks. The authors also demonstrate that the prediction accuracy of the semantic segmentation model trained from finite element (FE) simulation data is comparable with that of the model trained from experimental data, thus making their proposed machine learning approach cost-effective.
The article appears to be well researched and reliable in its content and claims, as it provides detailed information on each step of its proposed method and includes references to relevant studies in this field. Furthermore, it does not appear to contain any promotional content or partiality towards any particular method or technology. However, there are some points which could have been explored further in order to make the article more comprehensive and trustworthy. For example, while the authors mention potential risks associated with using X-ray computerized tomography (CT), they do not provide any details on how these risks can be mitigated or avoided when using this method for damage detection in composite structures. Additionally, while they discuss various existing NDE methods available for detecting defects inside composite structures, they do not provide an analysis on which method would be most suitable for a given application or scenario. Finally, while they present their own findings regarding their proposed machine learning approach for DIC measurement being cost-effective, they do not explore counterarguments or other possible solutions which may be more suitable in certain cases.